TransNet
Transparent Object Manipulation Through Category-Level Pose Estimation
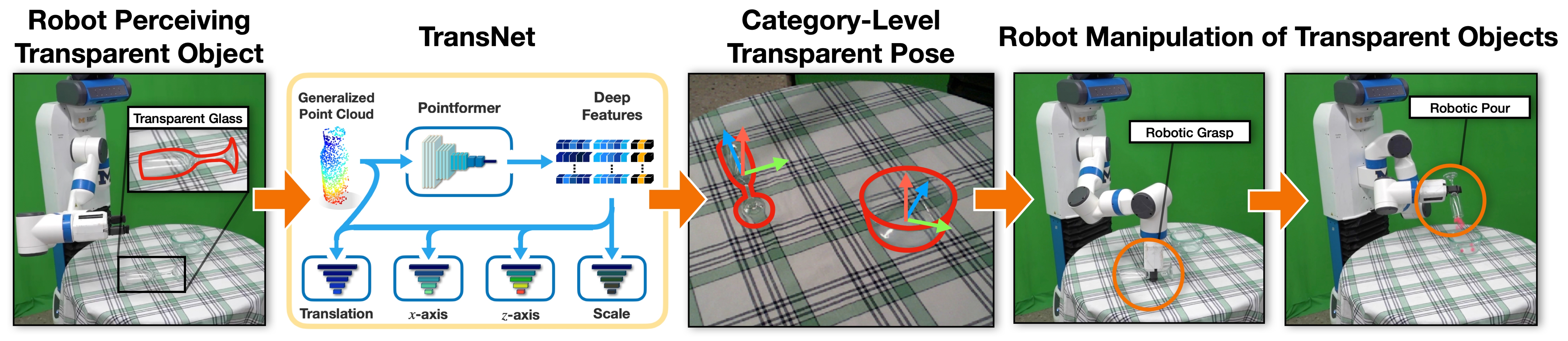
Transparent objects present multiple distinct challenges to visual perception systems. First, their lack of distinguishing visual features makes transparent objects harder to detect and localize than opaque objects. Even humans find certain transparent surfaces with little specular reflection or refraction, like glass doors, difficult to perceive. A second challenge is that the depth sensors typically used for opaque object perception cannot obtain accurate depth measurements on transparent objects due to their unique reflective properties. Stemming from these challenges, we observe that transparent object instances within the same category, such as cups, look more similar to each other than to ordinary opaque objects of that same category. Given this observation, the present paper explores the possibility of category-level transparent object pose estimation rather than instance-level pose estimation. We propose TransNet, a two-stage pipeline that estimates category-level transparent object pose using localized depth completion and surface normal estimation. TransNet is evaluated in terms of pose estimation accuracy on a recent large-scale transparent object dataset and compared to a state-of-the-art category-level pose estimation approach. Results from this comparison demonstrate that TransNet achieves improved pose estimation accuracy on transparent objects. Moreover, we use TransNet to build an autonomous transparent object manipulation system for robotic pick-and-place and pouring tasks.