ClearPose
Large-scale Transparent Object Dataset and Benchmark
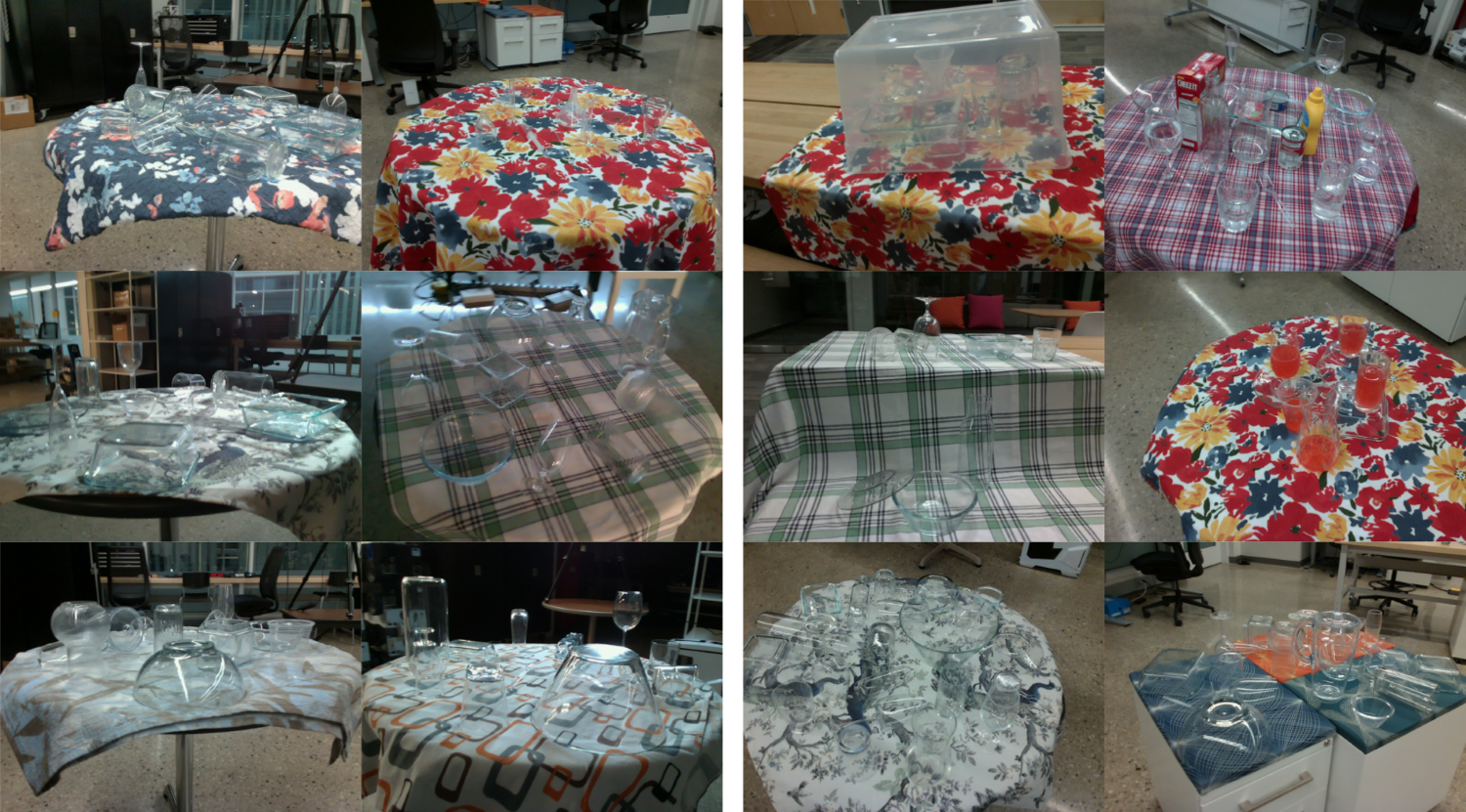
Transparent objects are ubiquitous in household settings and pose distinct challenges for visual sensing and perception systems. The optical properties of transparent objects leave conventional 3D sensors alone unreliable for object depth and pose estimation. These challenges are highlighted by the shortage of large-scale RGB-Depth datasets focusing on transparent objects in real-world settings. In this work, we contribute a large-scale real-world RGB-Depth transparent object dataset named ClearPose to serve as a benchmark dataset for segmentation, scene-level depth completion and object-centric pose estimation tasks. The ClearPose dataset contains over 350K labeled real-world RGB-Depth frames and 4M instance annotations covering 63 household objects. The dataset includes object categories commonly used in daily life under various lighting and occluding conditions as well as challenging test scenarios such as cases of occlusion by opaque or translucent objects, non-planar orientations, presence of liquids, etc. We benchmark several state-of-the-art depth completion and object pose estimation deep neural networks on ClearPose.